The existing AI craze is concentrated on generative AI innovations like ChatGPT, DALL-E, and Gemini that develop initial writing, images, computer system code, and even music. In medication, individuals are tossing GPT at every issue under the sun, evaluating it as a scribe that can assist medical professionals total medical recordsa chatbot that can use public health recommendations, and a tool to identify clients and advise treatment.
While a number of these applications are appealing, GPT is not the catch-all healthcare option individuals desire it to be. As an entire, AI has huge capacity to change medication. We can do so much more with it if we utilize the ideal AI tools for each job and thoroughly integrate them with existing clinical understanding.
What’s Missing from Our Current Conversation
In my laboratory at Boston University, we utilize AI to map the brain and much better comprehend conditions like autism and epilepsy. There are 2 huge things I think we’re missing out on in our rush to use generative AI in healthcare:
1) We require the best sort of information in the correct amounts.
Not every tool is ideal for every single job. GPT and other big language designs (LLMs) are text-based. A lot of medication relies on spatial or visual information like imaging scans, hereditary information, and photos that can’t be quickly boiled down to text. The AI I utilize in my research study, for instance, is more comparable to computer system vision designs than LLMs.
A 2nd concern is the quantity of information we have. Designs like GPT and DALL-E are trained on enormous caches of text and images pulled from the whole web. In lots of branches of medication, information is far more pricey and time extensive to gather. Running a single MRI expenses numerous dollars and can take an hour to finish. Not to point out the expenses to bring the client to an MRI center, personnel to run the MRI, and facilities to support the devices.
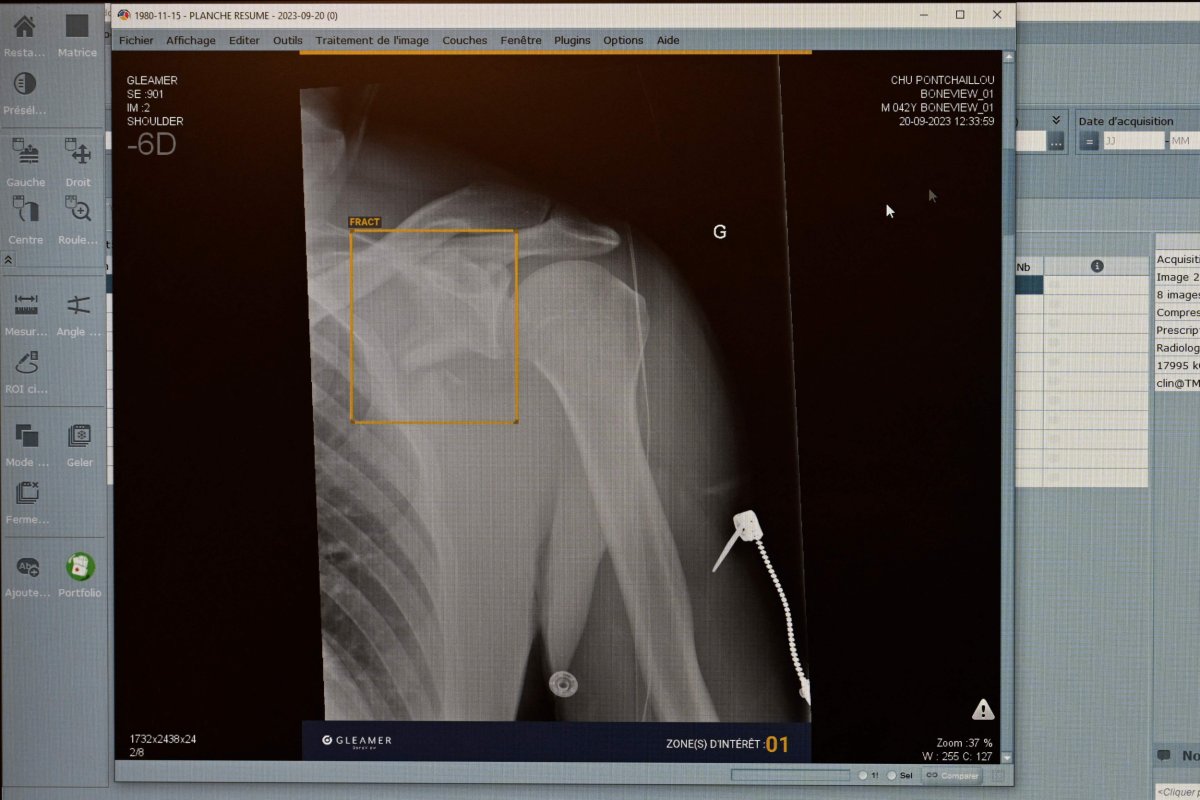
DAMIEN MEYER/AFP by means of Getty Images
We do not have a web’s worth of pictures of what various individuals’s brains appear like to train a design like GPT. Aggregating information throughout medical facilities has its own issues, for instance, by presenting predispositions that really aggravate efficiency. Rather, we depend on structured AI architectures and utilize existing biological and scientific understanding to form our designs.
2) We require to integrate information with existing clinical understanding.
In my laboratory, we established an algorithm that can identify epileptic seizures and identify where they are occurring in the brain. The algorithm utilizes an AI strategy called deep discovering to determine patterns in electroencephalography (EEG) information that reveal electrical activity in the brain. In screening, it beat existing advanced seizure detectors that do not utilize deep knowing.
The crucial distinction in between our work and existing tools is that we deal with the seizure as a developing procedure, generating what we understand about how seizure activity spreads in the brain, and utilize AI architectures that record this proliferation. This technique enables us to make robust forecasts from fairly little datasets.
Picture attempting to find out a language by plopping yourself down in a foreign nation– this is a rough approximation of how generative AI is trained. Structure existing science into our AI designs belongs to having somebody describe the alphabet and grammar guidelines to you initially, then setting you loose to absorb the language.
The Real Promise of AI in Medicine
The brain is noticeably complicated, with almost 100,000,000,000 nerve cells that user interface throughout trillions of neural connections. A single scan of somebody’s brain or sequencing of their genome consists of a big quantity of information. AI supercharges our capability to learn this information and acknowledge patterns.
Among our jobs utilizes biologically-inspired AI architectures to examine fMRI brain imaging and hereditary information and determine connections that may underlie inheritable neurological conditions like schizophrenia. In the past, researchers would concentrate on a couple of pre-identified brain locations and hereditary markers that they thought represented a specific condition. AI permits us to examine somebody’s whole brain and genome at the same time to find patterns we didn’t understand existed.
AI will transform medication in extraordinary methods. While generative AI will definitely have an effect, if we focus excessive on it, we will lose out on all the other methods AI can assist us comprehend the human brain and body.
Medical professionals, researchers, and funders should not be taken in by the buzz or sidetracked by fancy applications with minimal scientific worth. We need to keep our concentrate on utilizing the ideal AI tools for the task and structure on existing scientific understanding to develop really transformative science.
Dr. Archana Venkataraman is an associate teacher in Boston University’s College of Engineering, where she utilizes AI to study the brain and establish brand-new techniques to dealing with brain conditions like autism, epilepsy, and schizophrenia.
The views revealed in this short article are the author’s own.
Unusual Knowledge
Newsweek is devoted to tough traditional knowledge and finding connections in the look for commonalities.
Newsweek is devoted to tough traditional knowledge and finding connections in the look for commonalities.